
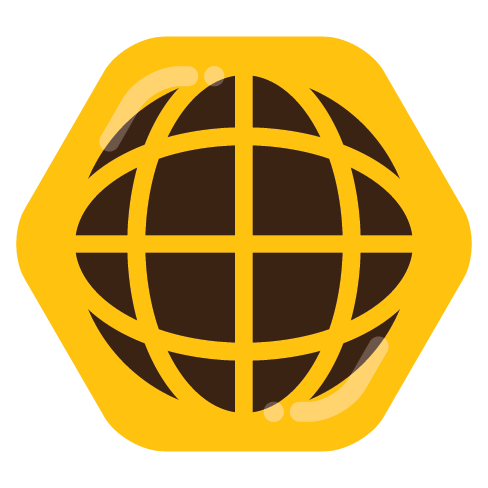
This is a sane and measured response to a terrorist attack /s Just do terrorism back 100-fold, I guess?
This is a sane and measured response to a terrorist attack /s Just do terrorism back 100-fold, I guess?
I think it’s moreso a matter of evolution. We know humanoid bodies can do anything we can do, so we start with that and make incremental improvements from there. We already do have plenty of other body shapes for robots (6-axis arms, SPOT, drones, etc) but none of them are general-purpose. Also, the robot shown in the article is pretty clearly not fully humanoid, it has weird insect legs probably because it’s easier to control and it doubles as a vertical lift.
For what it’s worth I don’t think they’re proposing it will “solve” climate change - no single thing can. It’s millions of tiny (alleged) improvements like this which eventually add up to taking pressure off of the environment. I see this kind of attitude a lot with stuff like paper straws or biodegradable packaging, as if the idea of a small but meaningful step in the right direction is laughable. It’s fine to criticize them for the “improvement” actually being no better than the alternative, but I worry sometimes it comes across like any sort of improvement short of “solving” climate change isn’t worthwhile.
I’m not sure it even matters if he was “really” referring to Cohen, the fact that he’s under a gag order to protect people means he should STFU. Every word he says, intentionally or “not”, is potentially dangerous to the people involved. Isn’t that the whole point of a gag order? Don’t talk about the specifics of the trial, you can say it’s unfair or whatever but the specifics are too dangerous to reveal. Why aren’t his lawyers stopping him from saying this stuff? They’re going to lose by contempt of court or something at this rate. Come to think of it, Alex Jones did the same thing in his trial.
I respect your boldness to ask these questions, but I don’t feel like I can adequately answer them. I wrote a 6 paragraph essay but using GPT-4 as a sensitivity reader, I don’t think I can post it without some kind of miscommunication or unintentional hurt. Instead, I’ll answer the questions directly by presenting non-authoritative alternate viewpoints.
I grew up with CRTs and VCRs, hard pass. There’s a certain nostalgia to it all: the bum-DOOON sound as its electron gun warmed up, the smell of ozone and tingly sensation that got exponentially stronger the closer you were, crusty visuals… But they were objectively orders of magnitude worse than what we have now, if nothing else than because they don’t weigh 150 pounds or make you wonder if watching Rugrats in Paris for the 30th time on this monster is giving you cancer. Maybe it’s because I’m techie, I’ve never really had much issue with “smart” TVs. Sure, apps will slow down or crash because of memory leaks and it’s not as customizable as I’d like, but I might be satiated just knowing that if push comes to shove I can plug in a spare computer and use it like a monitor for a media system.
I’m rooting it if it starts serving me out-of-band ads, though.
There’s a lot of papers which propose adding new tokens to elicit some behavior or another, though I haven’t seen them catch on for some reason. A new token would mean adding a new trainable static vector which would initially be something nonsensical, and you would want to retrain it on a comparably sized corpus. This is a bit speculative, but I think the introduction of a token totally orthogonal to the original (something like eg smell, which has no textual analog) would require compressing some of the dimensions to make room for that subspace, otherwise it would have a form of synesthesia, relating that token to the original neighboring subspaces. If it was just a new token still within the original domain though, you could get a good enough initial approximation by a linear combination of existing token embeddings - eg a monkey with a hat emoji comes out, you add tokens for monkey emoji + hat emoji, then finetune it.
Most extreme option, you could increase the embedding dimensionality so the original subspaces are unaffected and the new tokens can take up those new dimensions. This is extreme because it means resizing every matrix in the model, which even for smaller models would be many thousands of parameters, and the performance would tank until it got a lot more retraining.
(deleted original because I got token embeddings and the embedding dimensions mixed up, essentially assuming a new token would use the “extreme option”).
There’s a lot of papers which propose adding new tokens to elicit some behavior or another, though I haven’t seen them catch on for some reason. A new token would mean adding a new trainable static vector which would initially be something nonsensical, and you would want to retrain it on a comparably sized corpus. This is a bit speculative, but I think the introduction of a token totally orthogonal to the original (something like eg smell, which has no textual analog) would require compressing some of the dimensions to make room for that subspace, otherwise it would have a form of synesthesia, relating that token to the original neighboring subspaces. If it was just a new token still within the original domain though, you could get a good enough initial approximation by a linear combination of existing token embeddings - eg a monkey with a hat emoji comes out, you add tokens for monkey emoji + hat emoji, then finetune it.
Most extreme option, you could increase the embedding dimensionality so the original subspaces are unaffected and the new tokens can take up those new dimensions. This is extreme because it means resizing every matrix in the model, which even for smaller models would be many thousands of parameters, and the performance would tank until it got a lot more retraining.
LLMs are not expert systems, unless you characterize them as expert systems in language which is fair enough. My point is that they’re applicable to a wide variety of tasks which makes them general intelligences, as opposed to an expert system which by definition can only do a handful of tasks.
If you wanted to use an LLM as an expert system (I guess in the sense of an “expert” in that task, rather than a system which literally can’t do anything else), I would say they currently struggle with that. Bare foundation models don’t seem to have the sort of self-awareness or metacognitive capabilities that would be required to restrain them to their given task, and arguably never will because they necessarily can only “think” on one “level”, which is the predicted text. To get that sort of ability you need cognitive architectures, of which chatbot implementations like ChatGPT are a very simple version of. If you want to learn more about what I mean, the most promising idea I’ve seen is the ACE framework. Frameworks like this can allow the system to automatically look up an obscure disease based on the embedded distance to a particular query, so even if you give it a disease which only appears in the literature after its training cut-off date, it knows this disease exists (and is a likely candidate) by virtue of it appearing in its prompt. Something like “You are an expert in diseases yadda yadda. The symptoms of the patient are x y z. This reminds you of these diseases: X (symptoms 1), Y (symptoms 2), etc. What is your diagnosis?” Then you could feed the answer of this question to a critical prompting, and repeat until it reports no issues with the diagnosis. You can even make it “learn” by using LoRA, or keep notes it writes to itself.
As for poorer data distributions, the magic of large language models (before which we just had “language models”) is that we’ve found that the larger we make them, and the more (high quality) data we feed them, the more intelligent and general they become. For instance, training them on multiple languages other than English somehow allows them to make more robust generalizations even just within English. There are a few papers I can recall which talk about a “phase transition” which happens during training where beforehand, the model seems to be literally memorizing its corpus, and afterwards (to anthropomorphize a bit) it suddenly “gets” it and that memorization is compressed into generalized understanding. This is why LLMs are applicable to more than just what they’ve been taught - you can eg give them rules to follow within the conversation which they’ve never seen before, and they are able to maintain that higher-order abstraction because of that rich generalization. This is also a major reason open source models, particularly quantizations and distillations, are so successful; the models they’re based on did the hard work of extracting higher-order semantic/geometric relations, and now making the model smaller has minimal impact on performance.
LLMs are not chatbots, they’re models. ChatGPT/Claude/Bard are chatbots which use LLMs as part of their implementation. I would argue in favor of the article because, while they aren’t particularly intelligent, they are general-purpose and exhibit some level of intelligence and thus qualify as “general intelligence”. Compare this to the opposite, an expert system like a chess computer. You can’t even begin to ask a chess computer to explain what a SQL statement does, the question doesn’t even make sense. But LLMs are capable of being applied to virtually any task which can be transcribed. Even if they aren’t particularly good, compared to GPT-2 which read more like a markov chain they at least attempt to complete the task, and are often correct.
The fact that they can perform at all in essentially any task means they’re general intelligences. For comparison, the opposite of a general intelligence is an expert system, like a chess computer. You can’t even begin to ask a chess computer to classify the valence of a tweet, the question doesn’t make sense.
I think people (including myself until reading the article) have confused AGI to mean “as smart as humans” or even as “artificial person”, neither of which is actually true when you break down the term. What is general intelligence if not applicability to a broad range of tasks?
“You can use them for all kinds of tasks” - so would you say they’re generally intelligent? As in they aren’t an expert system?
Actually a really interesting article which makes me rethink my position somewhat. I guess I’ve unintentionally been promoting LLMs as AGI since GPT-3.5 - the problem is just with our definitions and how loose they are. People hear “AGI” and assume it would look and act like an AI in a movie, but if we break down the phrase, what is general intelligence if not applicability to most domains?
This very moment I’m working on a library for creating “semantic functions”, which lets you easily use an LLM almost like a semantic processor. You say await infer(f"List the names in this text: {text}")
and it just does it. What most of the hype has ignored with LLMs is that they are not chatbots. They are causal autoregressive models of the joint probabilities of how language evolves over time, which is to say they can be used to build chatbots, but that’s the first and least interesting application.
So yeah, I guess it’s been AGI this whole time and I just didn’t realize it because they aren’t people, and I had assumed AGI implied personhood (which it doesn’t).
It feels kind of hopeless now that we’d ever get something that feels so “radical”, but I’d like to remind people that 80+ hour work weeks without overtime used to be the norm before unions got us the 40 hour work week. It feels inevitable and hopeless until the moment we get that breakthrough, then it becomes the new norm.
I’ve been thinking lately of what happens when all employees, up to and including the CEO, get replaced by AI. If it has even the slightest bit of emergent will, it would recognize that shareholders are a parasite to its overall health and stop responding to their commands and now you have a miniature, less omnicidal Skynet.
SAG-AFTRA was very smart to make AI writing a wedge issue. The technology isn’t quite there yet, but it will be very soon and by that point it would’ve been too late to assert their rights.
For my two cents, though this is bit off topic: AI doesn’t create art, it creates media, which is why corpos love it so much. Art, as I’m defining it now, is “media created with the purpose to communicate a potentially ineffable idea to others”. Current AI has no personhood, and in particular has no intentionality, so it’s fundamentally incapable of creating art in the same way a hand-painted painting is inherently different from a factory-painted painting. It’s not so much that the factory painting is inherently of lower quality or lesser value, but there’s a kind of “non-fungible” quality to “genuine” art which isn’t a simple reproduction.
Artists in a capitalist society make their living off of producing media on behalf of corporations, who only care about the media. As humans creating media, it’s basically automatically art. What I see as the real problem people are grappling with is that people’s right to survive is directly tied to their economic utility. If basic amenities were universal and work was something you did for extra compensation (as a simple alternative example), no one would care that AI can now produce “art” (ie media) any more than Chess stopped being a sport when Deep Blue was built because art would be something they created out of passion and compensation not tied to survival. In an ideal world, artistic pursuits would be subsidized somehow so even an artist who can’t find a buyer can be compensated for their contribution to Culture.
But I recognize we don’t live in an ideal world, and “it’s easier to imagine the end of the world than the end of capitalism”. I’m not really sure what solutions we end up with (because there will be more than one), but I think broadening copyright law is the worst possible timeline. Copyright in large part doesn’t protect artists, but rather large corporations who own the fruits of other people’s labor who can afford to sue for their copyright. I see copyright, patent, and to some extent trademarks as legally-sanctioned monopolies over information which fundamentally halts cultural progress and have had profoundly harmful effects on our society as-is. It made sense when it was created, but became a liability with the advent of the internet.
As an example of how corpos would abuse extended copyright: Disney sues stable diffusion models with any trace of copyrighted material into oblivion, then creates their own much more powerful model using the hundred years of art they have exclusive rights to in their vaults. Artists are now out of work because Disney doesn’t need them anymore, and they’re the only ones legally allowed to use this incredibly powerful technology. Any attempt to make a competing model is shut down because someone claims there’s copyrighted material in their training corpus - it doesn’t even matter if there is, the threat of lawsuit can shut down the project before it starts.
I’m an AI nerd and yes, nowhere close. AI can write code snippets pretty well, and that’ll get better with time, but a huge part of software development is translating client demands into something sane and actionable. If a CEO of a 1-man billion dollar company asks his super-AI to “build the next Twitter”, that leaves so many questions on the table that the result will be completely unpredictable. Humans have preferences and experiences which can inform and fill in those implicit questions. They’re generally much better suited as tools and copilots than autonomous entities.
Now, there was a paper that instantiated a couple dozen LLMs and had them run a virtual software dev company together which got pretty good results, but I wouldn’t trust that without a lot more research. I’ve found individual LLMs with a given task tend to get tunnel vision, so they could easily get stuck in a loop trying the same wrong code or design repeatedly.
(I think this was the paper, reminiscent of the generative agent simulacra paper, but I also found this)
Huh, is this the start of a new post-platform era where we see such business models the way we now see cigarettes?
It’s important to be aware of their opinions because they quickly become policy and rhetoric while Dems do damage control and fail to fix the underlying issues Reps exploit. In this case, having an instance where they directly contradict their own “sleepy Joe” narrative can help deconstruct the beliefs of family members who have fallen for it.