
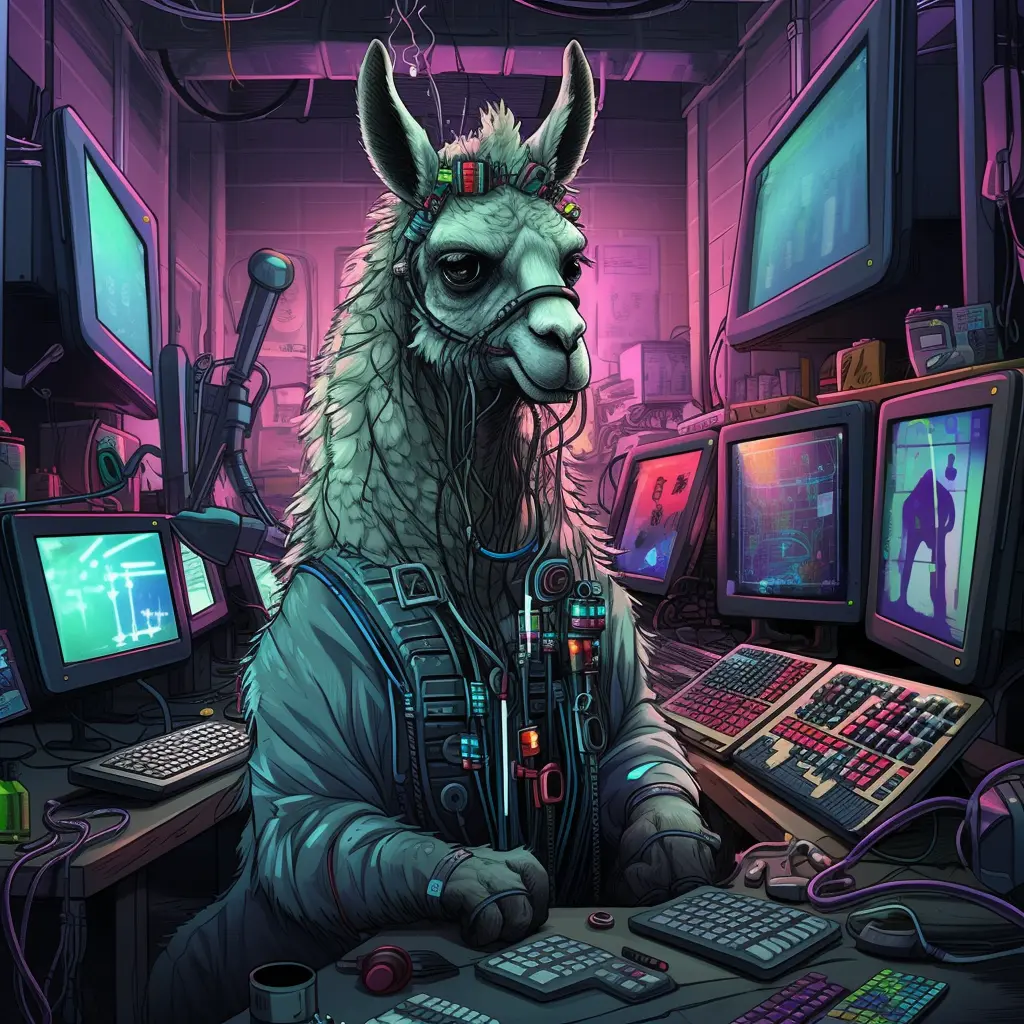
Don’t know OP and below is not aimed at him. But most people call stuff ‘unbiased’ if it’s aligned with their own biases. “Outsmarting” your own brain and self-awareness on meta level is really hard.
Don’t know OP and below is not aimed at him. But most people call stuff ‘unbiased’ if it’s aligned with their own biases. “Outsmarting” your own brain and self-awareness on meta level is really hard.
I think they have more than 700M customers.
I think they wouldn’t have a problem to negotiate custom license.
Ha! Just as I started writing my own thing for that. Will def take a look! :)
I doubt there’s any model there.
I doubt there is any model really… follow the trail, you’ll end up at a company founded by single person from India (who is founder of another company with a single app for collaborative drawing)… that at least doesn’t have any employees on LinkedIn…
And the founder looks like a relatively young person that most likely wouldn’t be even able to gather the required funding to have enough GPU compute for making model that’s better than gpt4 (or know how). I think that’s just a front for him trying to get some hype or funding.
It’s a source. But rarely synthetic benchmarks give you the whole picture. Plus those test sets are in the public, so there is some incentive for some people to game the system (and even without that those data sets most likely are already in the training data).
I’m seconding that. I’m actually amazed by how it performs, frequently getting similar or better answers than bigger models. I start to think that we do lose a lot with quantization from the bigger models…
You either do standardized benchmarks like that leader-board (which are useful but limited) or you have your application-specific benchmark. Most often the latter are very, very time&work consuming to do right. Evaluating NLP systems in general is very hard problem.
Some people use more powerful models to evaluate weaker ones. E.g., use GPT4 to evaluate output of llama. Depending on your task it might work well. I did recently an early version of experiment with around 20 models for text summarization, where GPT4 and I were evaluating summaries (on predefined scale, with predefined criteria of evaluation). I didn’t calculate any proper measure of inter annotator agreement yet, but looking at the evalas side by side it’s really high.
Or if you are just playing around, you just write/search for a post on reddit (or various LLM related discords) asking for best model for your task :D
Interesting. I’m using oobabooga and that never happened to me. I actually don’t recall it ever outputting anything but English…