
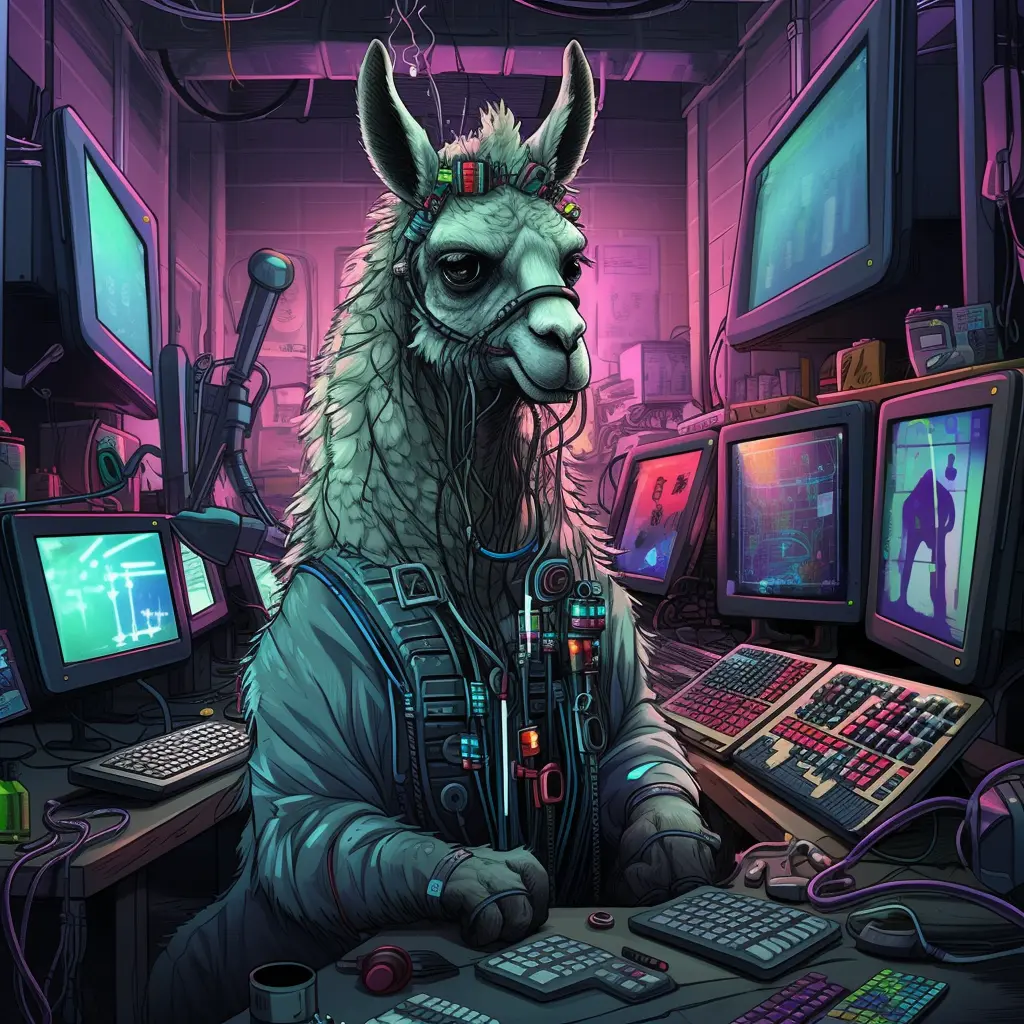
Temperature last is the assumed default of llama.cpp which means it is working.
Unfortunately the HF loader seems to have a bug with Min P in Ooba.
Temperature last is the assumed default of llama.cpp which means it is working.
Unfortunately the HF loader seems to have a bug with Min P in Ooba.
That doesn’t seem correct in the slightest.
Play with your sampler settings. The impact in creativity changes pretty significantly.
See this, for example:
The important elements are:
- Min P, which sets a minimum % relative to the top probability token. Go no lower than 0.03 for coherence at higher temps.
- Temperature, which controls how much the smaller probability options are considered and makes them more probable.
DynaTemp is still available in the test build.
I’m not sure which method is superior or anything yet, need more testing and opinions, but it looks promising because it scales well
exp-dynatemp-minp-latest branch had the changes pushed
Elon Musk has made it profitable
Applying Gaussian noise randomization to the logits with a gaussian deviation factor of 1.0 is totally coherent at top k = 1 (aka it’s picking the top token post randomization) on my Lora that I trained that I’m doing testing on and I haven’t seen repetition issues thus far. How might I test this? Like what are your best benchmark ideas?
Considering there’s an implementation of the cosine scheduler with warmup steps, is there any implementation of a scheduler that starts slow, then rapidly accelerates, and finally stabilizes to learn the subtle features (like a sigmoidal function?) To avoid starting too high in the first place.
So you’re saying my intuition isn’t wrong, necessarily, that slow training to learn the small subtle details could work as long as the dataset wasn’t *too* limited in scope?
https://huggingface.co/datasets/kalomaze/MiniSymposium-Demo-Dataset
Feel free to submit examples to the community tab
I am inclined to believe gpt4 since it consistently claims this across both the API and your comment… but I’m not sure
gpt4 is claiming this comment’s claim is wrong, but I can’t trust it blindly ofc, i’ll look into my initial claim to verify
> Multiple passes at lower learning rates isn’t supposed to produce different results.
Oh, I was wrong on this, then, my bad.
So would my interpretation be correct that this is essentially causing the overfitting to still happen, just significantly slower, and that a higher LR would work? The problem is at first the average loss tanked in the span of like a single epoch to near zero which overfit, but this LR didn’t have the same effect.
I posted that GitHub issue. That original Top K vs Top P graph wasn’t made by me, I can’t find the original source, but I made the Min P one and others.
What kind of sampler settings are you using? You can force models to get really out there in terms of creativity depending on what you use.
I am of the opinion that security through obscurity (of model weights) does not work.
The capabilities of these models would have to be consistently powerful beyond what the current state of the art is, and not just consistently, but by orders of magnitude to carry out the threats that have been proposed as pseudo-realistic risk.
Using your own compute instead of scraped GPT API keys when open models are at a state where their generalized performance is not directly comparable greatly diminishes the threat of bad actor risks. I’d maybe start to sweat if GPT4 was getting better instead of worse every time they do a rollout.
This is also another alignment paper that cites theoretical examples of biochemical terrorism. We live in a post-internet era where that type of information has already landed in the hands of the people who would be the most capable of carrying it out, but the post-internet era has consequentially also made those kinds of attacks much more difficult to carry out.
As the number of routes for possible attack vectors increases, the number of ways for that attack to be circumvented also increases.
I’m guessing GQA helped. Llama2 70b and 34b used Grouped Query Attention but it wasn’t used for Llama2 7/13b.
What frontends do you use?
Koboldcpp! Single exe, runs with very little dependency bloat, and is still blazing fast as long as you can offload the whole model.