
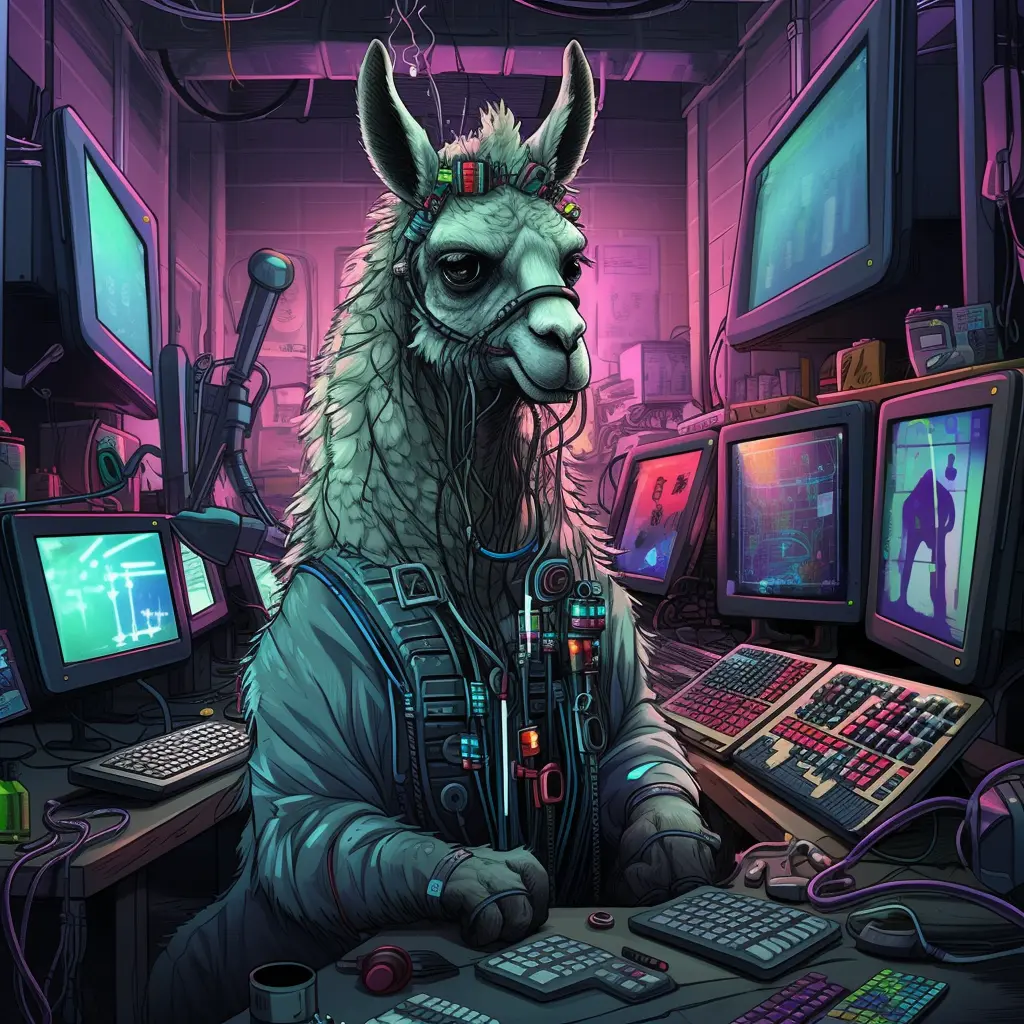
I’ve seen the “… beats GPT-4” enough times that now whenever I see a title that suggests a tiny model can compete with GPT-4 I see it as a negative signal; that the authors are bullshitting through some benchmarks or some other shenanigans.
It’s annoying because the models might be legitimately good models for being open and within their weight class but now you’ve put my brain in BS detecting mode and I can’t trust you’ve done good faith measurement anymore.
Some quotes I found on the pages:
“No! The model is not going to be available publically. APOLOGIES. The model like this can be misused very easily. The model is only going to be provided to already selected organisations.”
“[SOMETHING SPECIAL]: AIN’T DISCLOSING!🧟”
“Hallucinations: Reduced Hallucinations 8x compared to ChatGPT 🥳”
My guess: it’s just another merge like Goliath. At best it’s marginally better than a good 70B.
I can also “successfully build 220B model” easily with mergekit. Would it be good? Probably not.
The lab should write on their model card why should I not think it’s just bullshit. Not exactly the first mystery lab making big claims.